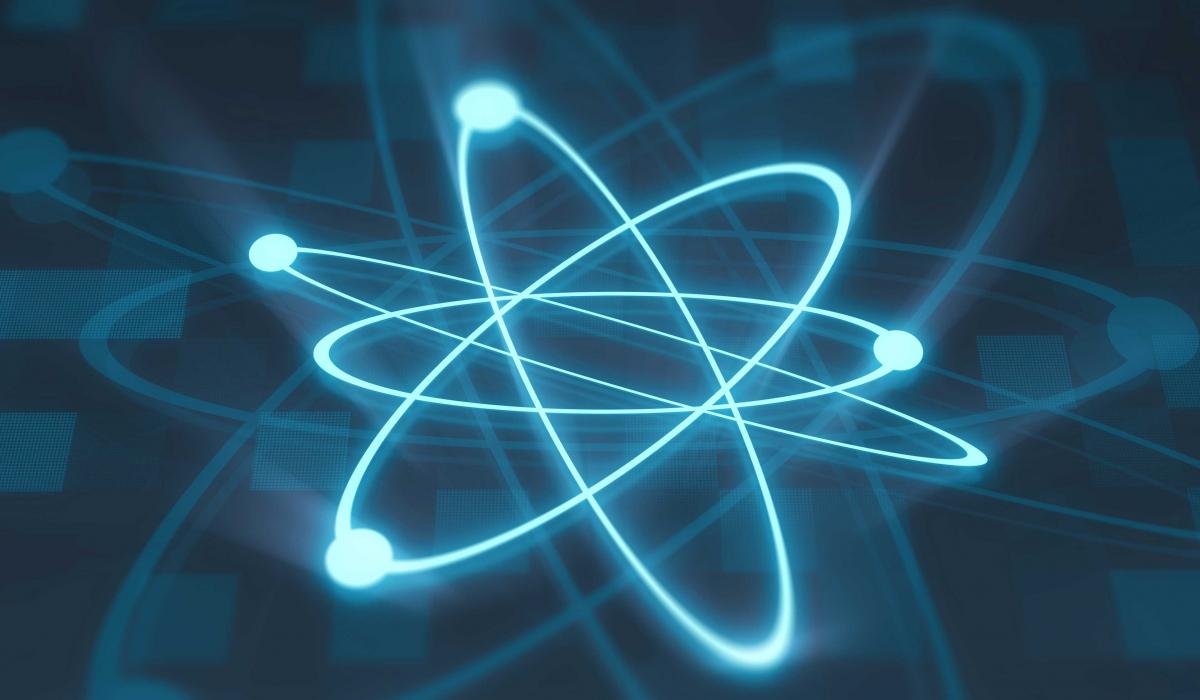
Adam Pecina
Molecular Modeling & Drug Discovery Lab, Istituto Italiano di Tecnologia, Genoa
Computational approaches have become an established and valuable component of pharmaceutical research. Computer-aided drug design (CADD) aims to reduce the time and cost of the drug development and also to bring deeper insight into the inhibitor binding to its target. The complexity of biological systems together with a need of proper description of non-covalent interactions involved in molecular recognition challenges the accuracy of commonly used molecular mechanical methods (MM). There is on the other side a growing interest of utilizing quantum mechanical (QM) methods in several stages of drug design thanks to increased computational resources. Quantum mechanics offers proper description of non-covalent interactions, covering quantum effects such as charge transfer and polarization. Moreover, it covers the vast of organic and inorganic chemical space without a need of ligand-dependent parameters. On the other hand, the exact solutions of the Schrödinger equation is limited to very small systems and protein-ligand interactions generally involve hundreds of atoms and are inherently very complex. The proper treatment thus must find the best balance between computational feasibility and accuracy.
Details of the implementations of QM approach in CADD drug discovery are well reviewed[1-5] and thus just a brief overview will be given in the workshop.
The lecture will mainly focus on an introduction of the new scoring function, which combines semi-empirical QM description of non-covalent interactions in the water phase. We will discuss the sampling and ranking power of the “SQM/COSMO” scoring function. We will go through several proof-of-concept studies to show how this approach increased fidelity of docking/scoring to identify native protein-ligand poses, outperforming standard scoring functions, surpassing the common limits and evaluating even small changes in the geometry of the ligand binding.[6,7]
We will also discuss its performance for metalloproteins and its ability to rank the different ligands according binding affinities, which is essential for hit-to-lead optimization and virtual screening. [8,9]
References:
[1] De Vivo, M. Front Biosci (Landmark Ed). 2011, 16, 1619.
[2] Mucs, D.; Bryce, R. A. Expert Opin Drug Dis 2013, 8, 263.
[3] Lepsik, M. et al. ChemPlusChem 2013, 78, 921.
[4] Merz, K. M. Accounts Chem Res 2014, 47, 2804.
[5] Crespo, A. et al. Curr Top Med Chem. 2017, 17, 2663.
[6] Pecina, A. et al. Chem. Comm. 2016, 52, 3312.
[7] Ajani, H. et al. ACS Omega 2017, 2, 4022.
[8] Pecina, A. et al. J. Chem. Inf. Model. 2017, 57, 127.
[9] Pecina, A. et al. ChemPhysChem 2018, 19, 873.